AI is often misunderstood. Discover the truth behind its capabilities and limitations, and how it’s reshaping the future of work and technology.
nanadwumor
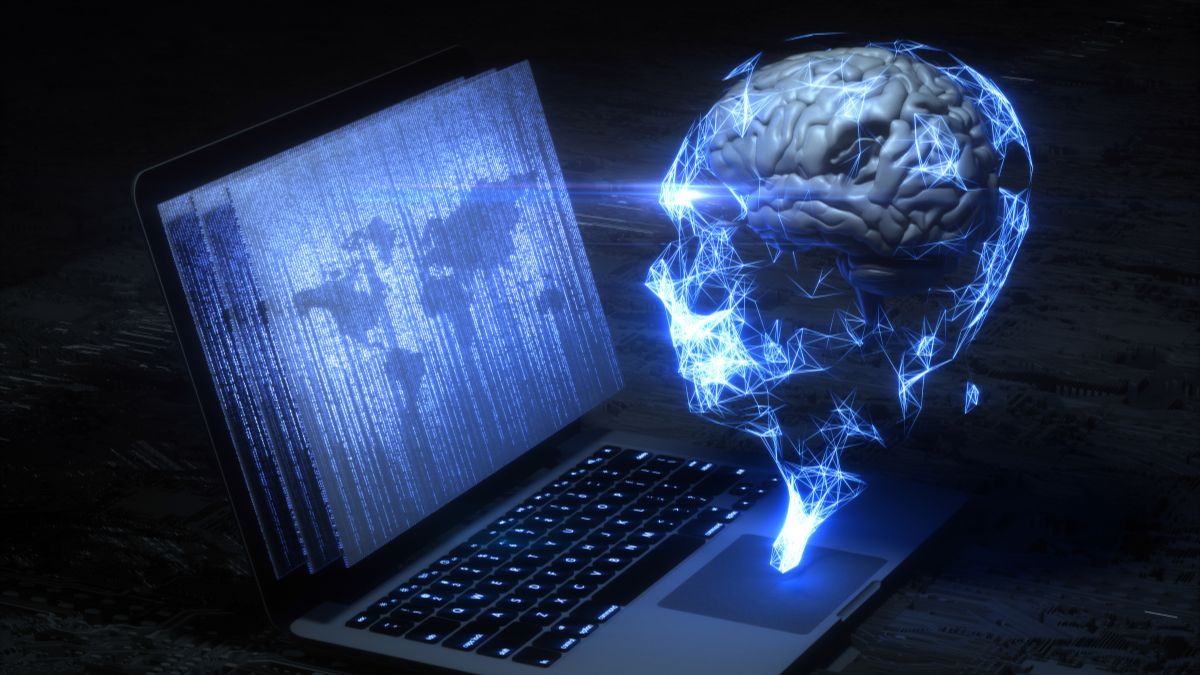
- AI can perform a wide range of tasks beyond creative activities, including supporting healthcare and finance with applications like data generation and risk modeling.
- AI will transform the job market by automating repetitive tasks and creating new roles that require skills in AI management and support.
- AI detection tools have limitations and can be fooled or misinterpreted, especially when detecting non-native English or modified content.
- Advances in on-device AI are bringing powerful AI capabilities to everyday devices, improving performance while reducing power consumption.
RECOMMENDED ARTICLES
Top 4 Ways on How to Connect Computer Keyboard to Your Phone
Find out how to type easily on your phone using PC keyboards. From connecting keyboards via OTG adapters to exploring the wireless wonders of Bluetooth, we've got you covered. Say goodbye to smartphone keyboard struggles and discover the ultimate typing solutions!...
How to Add iPhone-Like Dynamic Widgets to Your Android Phone
Do you want to know how to seamlessly integrate iPhone 14 Pro Dynamic Island experience interactive notch into your Android's touchscreen, complete with its ability to display notifications and adapt with your touch? You are in good hands. [Sassy_Social_Share...
WhatsApp Explores AI Sticker Generator in Latest Testing Phase
From Snapchat to Google Chrome, AI is revolutionizing tech giants, and now WhatsApp is joining the ranks. In a groundbreaking development, WhatsApp is testing AI-generated stickers for Android, transforming image descriptions into instant custom stickers....
Artificial intelligence is growing rapidly in today’s world. Some embrace it, while others feel unsure or fearful. Science fiction movies often create stories about AI taking over, but most concerns about AI come from real-world issues. Many worry their jobs might be taken by a low-cost Android powered by advanced AI and machine learning. Let me explain some common misconceptions and share the truth.
Generative AI is not limited to creative activities.
Generative AI is commonly associated with activities like creating art, writing stories, or composing music. However, its applications go further. In healthcare, it creates synthetic data for training and supports diagnosing diseases. In finance, it helps assess risks and simulates markets to improve investment planning.
AI will replace all jobs and leave us unemployed
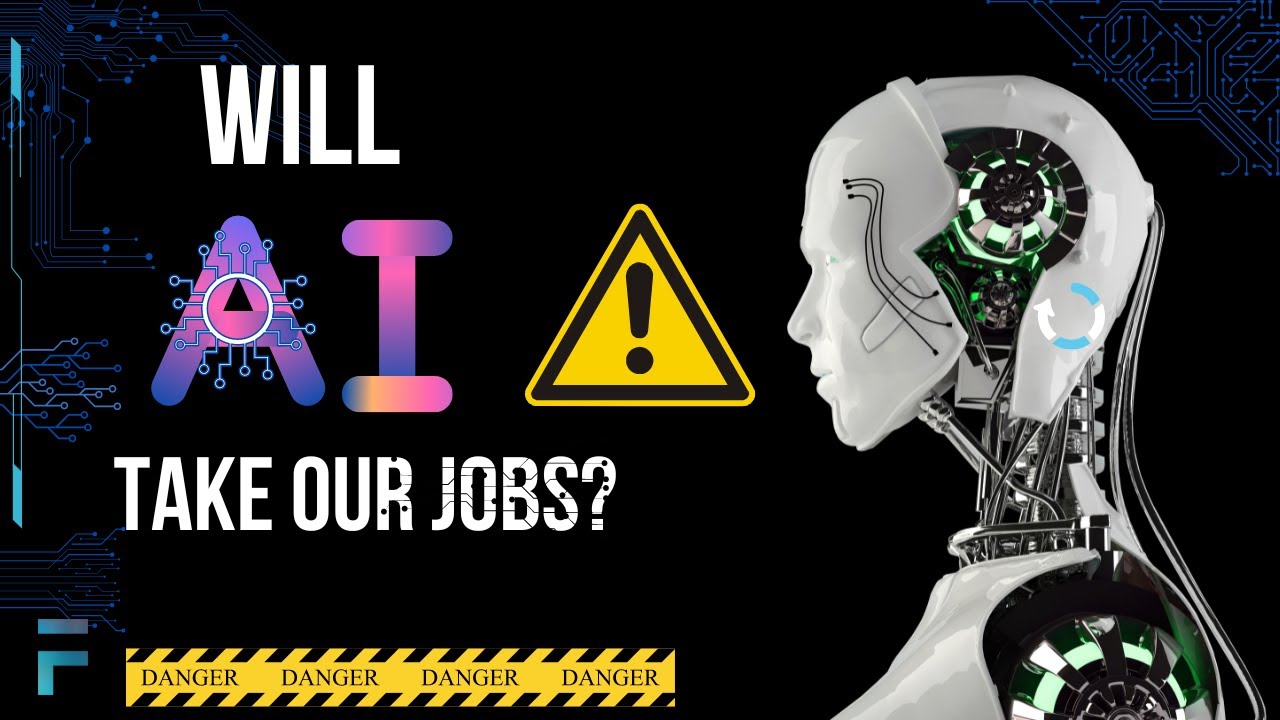
Technological progress often sparks concerns about job losses. AI performs specific, narrow tasks, but most jobs combine various interconnected duties. It streamlines repetitive work, freeing people to focus on tasks that need problem-solving and emotional skills.
AI will transform the job market by increasing baseline requirements and changing roles. In customer service, for instance, chatbots and virtual assistants manage simple tasks like balance checks or password resets. This reduces the need for entry-level workers.
Workers need to adapt by learning AI tools. Those who master these tools gain an advantage. AI will also open new opportunities, such as data annotators and compliance experts supporting its development and use.
AI detection tools CANNOT be fooled
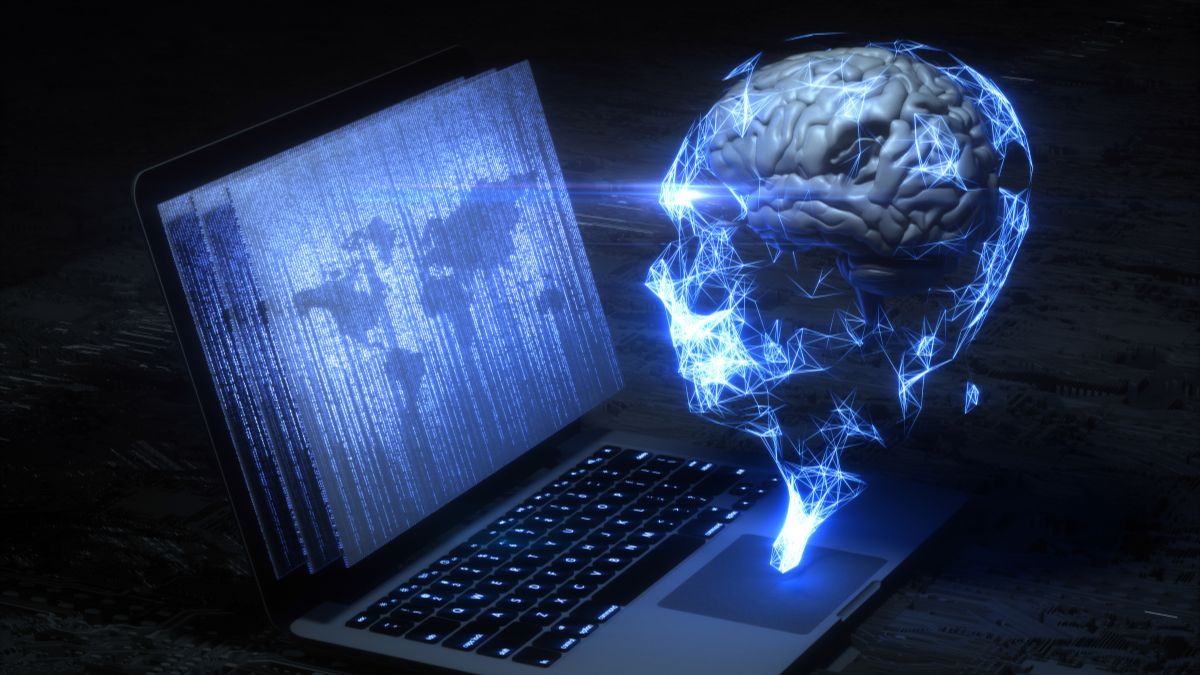
AI detection tools are still flawed. Studies reveal they often misjudge non-native English writing as AI-generated. This happens because detectors look for features in AI content that are also common in non-native writing. Techniques like altering content can further reduce their accuracy.
These tools function using models similar to the AI they aim to detect. They rely on two main metrics: perplexity and burstiness. Perplexity measures how predictable text is. AI content tends to be smooth but overly predictable, with low perplexity. Human writing is less predictable, showing higher perplexity with creativity and occasional mistakes. Burstiness measures sentence variety. AI text often lacks this, leading to uniformity, while human writing is more varied.
AI processing is limited to powerful machines.
AI is often thought to require supercomputers or cloud systems, making it seem impractical for regular devices. However, recent progress in on-device AI has introduced advanced capabilities to everyday tools. Companies like Arm are leading this change. The Cortex-X925 CPU, for instance, improves AI processing speed by 35% per clock cycle while using less power.
Arm’s Kleidi libraries help developers create AI solutions tailored for on-device use. Products like Google’s Gemini Nano and Apple Intelligence utilize these advancements to bring AI features directly to users.
It is still far from being a reality that AI will become sentient.
Artificial intelligence (AI) models, like ChatGPT and others based on the Transformer architecture, lack motivation, feelings, and life experiences. They do not have sentience, but they can mimic understanding by identifying patterns and formulating predictions. Even neural networks, which mirror some aspects of the brain’s structure, do not fully capture human cognition.
Moreover, the mechanisms of human consciousness and sentience are not well understood, complicating how we might define true machine sentience. This gap makes it challenging to establish clear criteria for recognizing genuine machine consciousness, even if it were theoretically possible. The differences between AI systems and the complexities of human consciousness are significant. For now, AI remains a tool, not a peer.
The decision-making process of AI systems is mysterious.
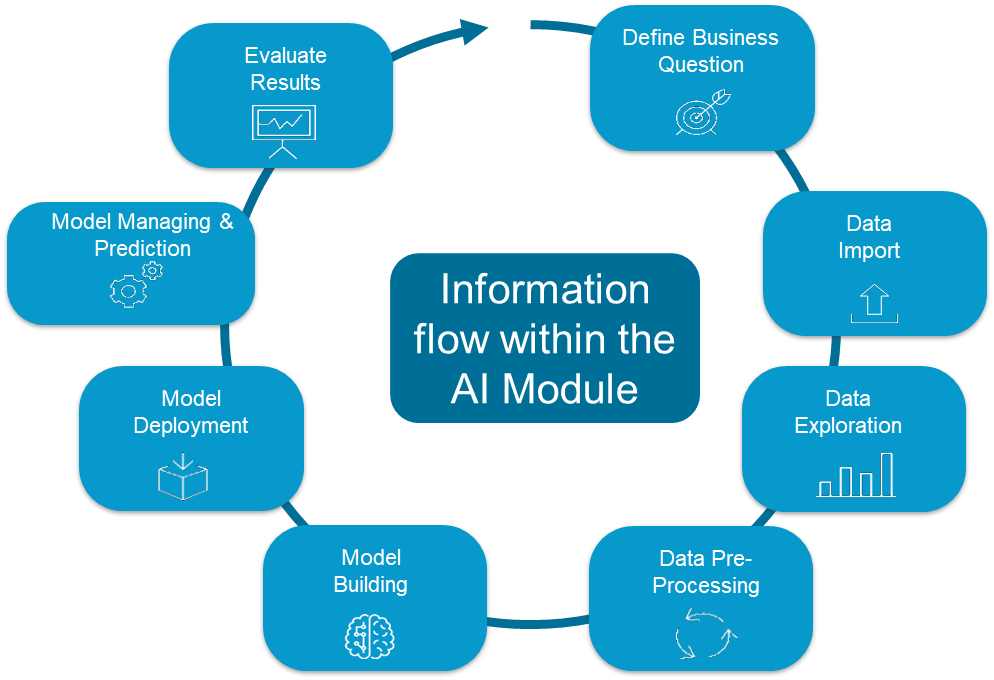
AI systems are not always black boxes. Explainable AI, sometimes referred to as white or glass box AI, and black box AI are the two main varieties. Black box systems’ decisions are difficult to understand because of their opaque processes. For instance, if a black box AI denies a business loan application, users would benefit from understanding the rationale behind the decision to potentially appeal. Researchers are working on techniques to enhance transparency in black box AI, such as:
- Finding features that have an effect on outputs (feature importance).
- Providing explanations for specific predictions (local explanations).
- Extracting human-readable rules from learned patterns (rule extraction).
- Visualizing internal model processes (visualization techniques).
Another term for AI is machine learning.

Machine learning and artificial intelligence (AI) are two separate but related areas of computer science that are frequently confused. The goal of artificial intelligence (AI) is to create machines that can reason, solve problems, and process language like a human. Developing algorithms that learn from data and generate predictions based on that learning is the focus of machine learning, a branch of artificial intelligence.
While machine learning plays a significant role in the development of AI, not all AI depends exclusively on it. Logic programming and rule-based systems are two methods that allow intelligent behavior without depending on data-driven learning. Similar to AI, machine learning can be used to power systems that offer insights and automation, even if they lack “intelligence.”
AI is biased and unfair by nature.
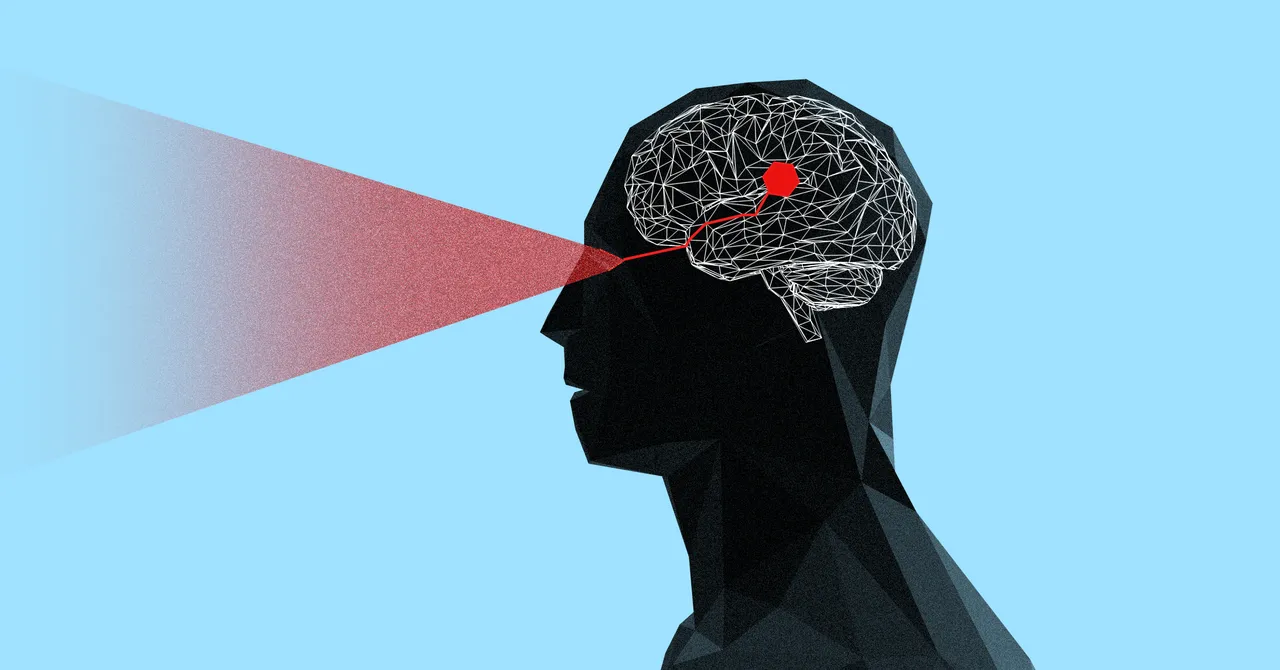
The idea that AI is essentially biased is frequently the result of misconceptions about how it works. AI bias primarily arises from the data used during training. For instance, if a recruitment tool learns from historical hiring data that contains gender or racial biases, it may replicate these patterns in its recommendations.
However, not all AI systems are biased. They can be retrained or adjusted to reduce bias and promote fair outcomes. AI can reduce human bias in decision-making by carefully choosing and preprocessing training data and putting in place systems to identify and address biases.
While machine learning plays a significant role in the development of AI, not all AI depends exclusively on it. Logic programming and rule-based systems are two methods that allow intelligent behavior without depending on data-driven learning. Similar to AI, machine learning can be used to power systems that offer insights and automation, even if they lack “intelligence.”
Join Our Telegram Group
Join Our WhatSapp Group
You May Also Like…
Top 4 Ways on How to Connect Computer Keyboard to Your Phone
Find out how to type easily on your phone using PC keyboards. From connecting keyboards via OTG...
How to Add iPhone-Like Dynamic Widgets to Your Android Phone
Do you want to know how to seamlessly integrate iPhone 14 Pro Dynamic Island experience...
WhatsApp Explores AI Sticker Generator in Latest Testing Phase
From Snapchat to Google Chrome, AI is revolutionizing tech giants, and now WhatsApp is joining the...
0 Comments